Checking for non-preferred file/folder path names (may take a long time depending on the number of files/folders) ...
This resource contains some files/folders that have non-preferred characters in their name. Show non-conforming files/folders.
This resource contains content types with files that need to be updated to match with metadata changes. Show content type files that need updating.
Authors: |
|
|
---|---|---|
Owners: |
|
This resource does not have an owner who is an active HydroShare user. Contact CUAHSI (help@cuahsi.org) for information on this resource. |
Type: | Resource | |
Storage: | The size of this resource is 1.6 GB | |
Created: | Oct 15, 2019 at 2:56 p.m. (UTC) | |
Last updated: | Feb 11, 2020 at 8:26 p.m. (UTC) (Metadata update) | |
Published date: | Jan 08, 2020 at 9:27 p.m. (UTC) | |
DOI: | 10.4211/hs.1a116e5460e24177999c7bd6f8292421 | |
Citation: | See how to cite this resource | |
Content types: | Single File Content Geographic Feature Content |
Sharing Status: | Published |
---|---|
Views: | 3916 |
Downloads: | 388 |
+1 Votes: | 1 other +1 this |
Comments: | No comments (yet) |
Abstract
SENSEmap-USGLB, the Spatially Explicit Nutrient Source Estimate map for the United States Great Lakes Basin, estimates inputs to the landscape from seven sources of nitrogen and six sources of phosphorus at 30 meter resolution for an average year during the 2008-2015 period. SENSEmap uses statistical and machine learning methods to estimate nutrient inputs using remotely sensed data, government records, and literature values. The sources include atmospheric deposition, chemical agricultural fertilizer, chemical nonagricultural fertilizer, manure, septic tanks, nitrogen fixation from legumes, and point sources. This resource includes 30 meter maps of each source along with corresponding watershed summaries at the Hydrologic Unit Code 12 (HUC12) and HUC8 levels, as defined in the USGS 2014 Watershed Boundary Dataset. Watershed summaries include total nitrogen and phosphorus in kg/yr, area normalized watershed inputs in kg/ha/yr, percent contribution of each source individually, and the percent contributions of combined agricultural sources and non-agricultural sources. Single-year per crop estimates of total nitrogen fixation inputs are also included. The values provided represent an average nutrient input in kg/ha/yr over the 2008-2015 period, generated from a single model realization. SENSEmap may be used to quantify nutrient inputs within nutrient budgets, process-based models, and water quality health indicators. SENSEmap estimates are not loads to groundwater, streams, or lakes and do not include nutrient exports due to harvest, denitrification, or other processes. SENSEmap is a regional-scale estimate, produced at fine resolution, and includes stochastic processes for certain inputs. As such, it should not be used for field level assessments, or where precise knowledge of local inputs is of key concern. SENSEmap-USGLB is described in full detail in the manuscript and supporting information of Hamlin et al.'s (2020) “Spatially Explicit Nutrient Source Estimate Map (SENSEmap): Quantifying Landscape Nutrient Inputs With Spatially Explicit Nutrient Source Estimate Maps in Journal of Geophysics: Biogeosciences (https://doi.org/10.1029/2019JG005134)
Subject Keywords
Coverage
Spatial
Temporal
Start Date: | |
---|---|
End Date: |
Content
readme.md
SENSEmap-USGLB: Nitrogen and Phosphorus Inputs
Authors: Quercus F. Hamlin, Anthony D. Kendall, Sherry L. Martin, Henry D. Whitenack, Jacob A. Roush, Bailey A. Hannah, David W Hyndman
Data Provided as a Resource for: Hamlin, QF, Kendall, AD, Martin, SL, Whitenack, HD, Roush, JA, Hannah, BA, Hyndman, DW. (2020). “Quantifying Landscape Nutrient Inputs With Spatially Explicit Nutrient Source Estimate Maps.” Journal of Geophysical Research: Biogeosciences. 10.1029/2019JG005134
Abstract: SENSEmap-USGLB, the Spatially Explicit Nutrient Source Estimate map for the United States Great Lakes Basin, estimates inputs to the landscape from seven sources of nitrogen and six sources of phosphorus at 30 meter resolution for an average year during the 2008-2015 period. SENSEmap uses statistical and machine learning methods to estimate nutrient inputs using remotely sensed data, government records, and literature values. The sources include atmospheric deposition, chemical agricultural fertilizer, chemical nonagricultural fertilizer, manure, septic tanks, nitrogen fixation from legumes, and point sources. This resource includes 30 meter maps of each source along with corresponding watershed summaries at the Hydrologic Unit Code 12 (HUC12) and HUC8 levels, as defined in the USGS 2014 Watershed Boundary Dataset. Watershed summaries include total nitrogen and phosphorus in kg/yr, area normalized watershed inputs in kg/ha/yr, percent contribution of each source individually, and the percent contributions of combined agricultural sources and non-agricultural sources. Single-year per crop estimates of total nitrogen fixation inputs are also included. The values provided represent an average nutrient input in kg/ha/yr over the 2008-2015 period, generated from a single model realization. SENSEmap may be used to quantify nutrient inputs within nutrient budgets, process-based models, and water quality health indicators. SENSEmap estimates are not loads to groundwater, streams, or lakes and do not include nutrient exports due to harvest, denitrification, or other processes. SENSEmap is a regional-scale estimate, produced at fine resolution, and includes stochastic processes for certain inputs. As such, it should not be used for field level assessments, or where precise knowledge of local inputs is of key concern. SENSEmap-USGLB is described in full detail in the manuscript and supporting information of Hamlin et al.'s (2020) “Quantifying Landscape Nutrient Inputs With Spatially Explicit Nutrient Source Estimate Maps” in Journal of Geophysics: Biogeosciences (10.1029/2019JG005134).
Using SENSEmap-USGLB:
Intended Use: SENSEmap-USGLB provides estimates of total nitrogen and total phosphorus inputs to the landscapes produced at 30 meter resolution. It is suitable for watershed and regional scale work where precise knowledge of local inputs is not important. It is not intended for field or farm level assessments.
Files Included:
- SENSEmap_USGLB_Sources.zip: Archive containing SENSEmap TN and TP nutrient sources in tif format. Point sources are included as shapefile. When uncompressed, the archive is 59.6 GB.
- SENSEmap_USGLB_HUC12: Shapefile of HUC12 watershed SENSEmap summaries.
- SENSEmap_USGLB_HUC8: Shapefile of HUC8 watershed SENSEmap summaries.
- SENSEmap_USGLB_Crop_Annual_NFixation.zip: Archive containing per crop annual N fixation.
- SENSEmap_Codebook.csv: Column names for HUC summaries (Column names can also be found in shapefile XML metadata).
Opening Files: SENSEmap nutrient sources rasters provided are zipped under high compression as tifs. Vector files are provided as shp. We recommend using an archive program like 7zip (free) to extract them. It may take up to 20 minutes to extract. If using in ArcGIS, pyramids should be built to view the rasters. In QGIS, if using a stretched color scheme please change stretch settings to min-max, rather than 2%-98%. Viewing at 2%-98% will produce a scale from zero to zero and the map will not display for some rasters. We recommend classifying rasters for better viewing.
Metadata: All rasters and shapefiles include metadata providing relevant citations and descriptions in each XML file. Shapefiles for HUC12 and HUC8 summaries include field descriptions in metadata. Field descriptions can also be downloaded as a csv within the Hydroshare resource. Files were processed in ArcGIS Desktop 10.4 and ArcGIS Pro 2.2.
Projection: SENSEmap uses the NAD 1983 Albers Great Lakes Albers projection (EPSG: 3174).
Resolution: 30 meter, HUC12 watershed, HUC8 watershed
Time Period: SENSEmap provides nutrient inputs for an average year during 2008-2015. Data sources used to build SENSEmap span this period.
Methods:
Full methodological description can be found in the accompanying manuscript and supporting information (10.1029/2019JG005134). Here, we briefly describe key datasets and methods used to derive SENSEmap for each nutrient source.
- Atmospheric Deposition (P): Interpolated using kriging of 98 P deposition sites across the eastern and midwest United States and southern portions of Canada. Sites were identified using an extensive literature review. A spreadsheet of these sites and studies can be found in Hamlin et al. (2020)’s Supporting Information. Wet and dry values were both converted and included, producing a sum of total phosphorus.
Atmospheric Deposition (N): Resampled and derived from 2008-2015 average of National Atmospheric Deposition Program (NADP) Total Deposition Science Committee (TDEP) product for total N deposition (wet+dry). Retrieved from ftp.epa.gov/castnet/tdep June 15, 2019. Described in Schwede and Lear (2014).
- Chemical Agricultural Fertilizer: Chemical agricultural fertilizer inputs were calculated based on spatially disaggregating USGS estimate of TN and TP agricultural fertilizer at the county level (Brakebill & Gronberg, 2017). Spatial disaggregation was performed with boosted regression trees (BRT) using crop type, soil texture, latitude, and county cropland area. Crop type was determined based on the USDA Cropland Data Layer (CDL).
- Chemical NonAgricultural Fertilizer: Chemical nonagricultural fertilizer inputs were calculated based on spatially disaggregating USGS estimates of TN and TP nonagricultural fertilizer at the county level (Brakebill & Gronberg, 2017). Disaggregation methods were based on census tract population, golf course locations, and impervious surface from the National Land Cover Database 2011.
- Manure: Manure was calculated using USDA 2012 Agricultural Census data on animal inventories at the county level. Farms were pseudo-randomly placed based on assumptions about land use and manure disposal. More details on farm placement can be found within the manuscript and supporting information Section Test S1.4. N and P loading rates were derived from literature, primarily USDA NRCS values. Because farms are pseudo-randomly placed, we advise against the use of SENSEmap for field or farm scale analysis.
- Nitrogen Fixation from Legumes: Nitrogen fixation from legumes are calculated based on soil organic matter, local N additions, and USDA Agricultural Census derived yields for soybeans, dry beans, alfalfa, and other hay. Calculations were based on equations set forth in Meisinger & Randall (1991). Due to the 2008-2015 average time period, we took into account the crop rotation at each pixel and scaled total N fixation to match the amount of years as an N fixing crop. Pixel totals were also adjusted based on the area of crops in the Ag Census, as there were more pixels labeled by CDL than reported area. Thus, the final N Fixation source map in SENSEmap is not representative of a single year of an N fixing crop, but rather an average N fixation input over the 2008-2015 time period. We have also included per-crop N fixation estimates for a single year of that crop.
- Point Sources: Point sources are provided as a point shapefile. Point sources were calculated from the EPA Discharge Monitoring Report (cfpub.epa.gov/dmr/), accessed in June 2017, which has reports for all NPDES permit-holding establishments. Units were converted to TN. The geometric mean of 2008-2015 was used.
- Septic Systems: Septic system locations were estimated using drinking water wells, population density, and distance from wastewater treatment plants. Inputs represent the TN and TP load to the septic tank, NOT the TN or TP load to groundwater after being processed by the system.
Notes on the N Fixation Annual Per-Crop files: The separate archive for N Fixation includes annual per-crop estimates of N fixation. These represent total N fixation in a single year planted. The total N Fixation map within the SENSEmap sources archive includes a crop-rotation adjusted total N fixation, thus lowering the values. If you are comparing SENSEmap to a single year N input, please compare to these per-crop files.
Works Cited
Brakebill, J., & Gronberg, J. (2017). County-Level Estimates of Nitrogen And Phosphorus from Commercial Fertilizer for the Conterminous United States, 1987-2012. 10.5066/F7H41PKX
Homer, C. G., Dewitz, J. A., Yang, L., Jin, S., Danielson, P., Xian, G., … Megown, K. (2015). Completion of the 2011 National Land Cover Database for the conterminous United States-Representing a decade of land cover change information. Photogrammetric Engineering and Remote Sensing, 81(5), 345–354. 10.14358/PERS.81.5.345
Meisinger, J. J., & Randall, G. W. (1991). Estimating Nitrogen Budgets for Soil-Crop Systems. In R. F. Follett, D. R. Keeney, & R. M. Cruse (Eds.), Managing nitrogen for groundwater quality and farm profitability (pp. 85–124). Madison, WI: Soil Science Society of America. 10.2136/1991.managingnitrogen.c5
Schwede, D. B., & Lear, G. G. (2014). A novel hybrid approach for estimating total deposition in the United States. Atmospheric Environment, 92, 207–220. 10.1016/j.atmosenv.2014.04.008
Data Services
Related Resources
This resource is referenced by | Hamlin, Q. F., Kendall, A. D., Martin, S. L., Whitenack, H. D., Roush, J. A., Hannah, B. A., & Hyndman, D. W. (2020). Quantifying landscape nutrient inputs with spatially explicit nutrient source estimate maps. Journal of Geophysical Research: Biogeosciences, 125, e2019JG005134. https://10.4211/10.1029/2019JG005134 |
The content of this resource is derived from | Homer, C. G., Dewitz, J. A., Yang, L., Jin, S., Danielson, P., Xian, G., … Megown, K. (2015). Completion of the 2011 National Land Cover Database for the conterminous United States-Representing a decade of land cover change information. Photogrammetric Engineering and Remote Sensing, 81(5), 345–354. |
The content of this resource is derived from | Schwede, D. B., & Lear, G. G. (2014). A novel hybrid approach for estimating total deposition in the United States. Atmospheric Environment, 92, 207–220. https://doi.org/10.1016/j.atmosenv.2014.04.008 |
The content of this resource is derived from | USDA National Agricultural Statistics Service. Cropland Data Layer 2008-2015. Washington, D.C.: USDA-NASS. Retrieved from https://nassgeodata.gmu.edu/CropScape/ |
The content of this resource is derived from | USDA Natural Resources Conservation Service (NRCS) (2017). Gridded Soil Survey Geographic (gSSURGO) Database: User Guide, version 2.2. Accessed 9/11/2017. |
The content of this resource is derived from | USDA National Agricultural Statistics Service. (2012). Census of Agriculture. |
The content of this resource is derived from | USEPA. (2010). Clean Air Status and Trends Network (CASTNET). Retrieved from https://www.epa.gov/castnet |
The content of this resource is derived from | USEPA. (2017). Discharge Monitoring Report (DMR) Pollutant Loading Tools. Retrieved July 10, 2017, from https://cfpub.epa.gov/dmr/ |
The content of this resource is derived from | US Census Bureau. (2010). US 2010 Census. |
The content of this resource is derived from | Brakebill, J., & Gronberg, J. (2017). County-Level Estimates of Nitrogen And Phosphorus from Commercial Fertilizer for the Conterminous United States, 1987-2012. https://doi.org/doi.org/10.5066/F7H41PKX |
Credits
Funding Agencies
This resource was created using funding from the following sources:
Agency Name | Award Title | Award Number |
---|---|---|
NASA | Quantifying How Global Change and Land Use Legacies Affect Ecosystem Processes at the Land Water Interface Across the Great Lakes Basin | 80NSSC17K0262 |
NOAA | Empowering Communities with Online Action Planning Tools: Tipping Points and Indicators for Improving Water Quality across the Great Lakes | NA12OAR4320071 |
NASA | Linking Remote Sensing and Process-based Models to Better Understand the Influence of Land Use and Climate Changes on Great Lakes Coastal Wetlands | NNX11AC72G |
USDA NIFA | Developing and promoting water-, nutrient-, and climate-smart technologies to help agricultural systems adapt to climate and societal change | 2015-68007-23133 |
How to Cite
This resource is shared under the Creative Commons Attribution CC BY.
http://creativecommons.org/licenses/by/4.0/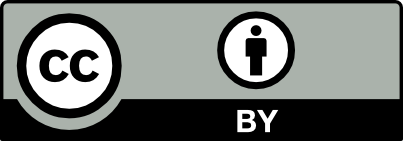
Comments
There are currently no comments
New Comment