Checking for non-preferred file/folder path names (may take a long time depending on the number of files/folders) ...
This resource contains some files/folders that have non-preferred characters in their name. Show non-conforming files/folders.
This resource contains content types with files that need to be updated to match with metadata changes. Show content type files that need updating.
Authors: |
|
|
---|---|---|
Owners: |
|
This resource does not have an owner who is an active HydroShare user. Contact CUAHSI (help@cuahsi.org) for information on this resource. |
Type: | Resource | |
Storage: | The size of this resource is 12.9 MB | |
Created: | Oct 21, 2020 at 4:25 p.m. (UTC) | |
Last updated: | Oct 27, 2020 at 4:16 p.m. (UTC) | |
Citation: | See how to cite this resource |
Sharing Status: | Public |
---|---|
Views: | 1893 |
Downloads: | 90 |
+1 Votes: | Be the first one to this. |
Comments: | No comments (yet) |
Abstract
Accurate rainfall-runoff modelling is particularly challenging due to complex nonlinear relationships between various factors such as rainfall characteristics, soil properties, land use, and temporal lags. Recently, with improvements to computation systems and resources, data-driven models have shown good performances for runoff forecasting. However, the relative performance of common data-driven models using small temporal resolutions is still unclear. This study presents an application of data-driven models using artificial neural network, support vector regression and long-short term memory approaches and distributed forcing data for runoff predictions between 2010 to 2019 in the Russian River basin, California, USA. These models were used to predict hourly runoff with 1 – 6 hours of lead time using precipitation, soil moisture, baseflow and land surface temperature datasets provided from the North American Land Data Assimilation System. The predicted results were evaluated in terms of seasonal and event-based performance using various statistical metrics. The results showed that the long-short term memory and support vector regression models outperforms artificial neural network model for hourly runoff forecasting, and the predictive performance of the models was greater during the wet seasons compared to the dry seasons. In addition, a comparison of the data-driven model results with the National Water Model, a fully distributed physical-based hydrologic model, showed that the long-short term memory and support vector regression models provide comparable performance. The results demonstrate that data-driven models for hourly runoff forecasting are sufficiently predictive and useful in areas where observation systems are not available.
Subject Keywords
Coverage
Spatial
Temporal
Start Date: | |
---|---|
End Date: |
Content
readme.txt
Data-Driven Approaches for Runoff Prediction using Distributed Data Metadata October 2020 There are three files available for download: 1) Observed_Runoff_11467000.csv - Hourly observed runoff at USGS station (11467000; 2010 - 2019) 2) Predicted_Runoff_11467000.csv - Predicted runoff from data-driven models (For test period, 2017 - 2019) 3) Input_Data.csv - Normalized input datasets of models (Training : 2010 - 2016, Test : 2017 - 2019)
How to Cite
This resource is shared under the Creative Commons Attribution CC BY.
http://creativecommons.org/licenses/by/4.0/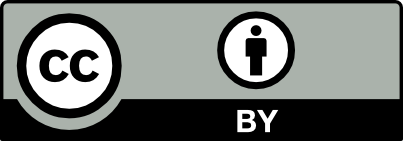
Comments
There are currently no comments
New Comment