Checking for non-preferred file/folder path names (may take a long time depending on the number of files/folders) ...
This resource contains some files/folders that have non-preferred characters in their name. Show non-conforming files/folders.
This resource contains content types with files that need to be updated to match with metadata changes. Show content type files that need updating.
Authors: |
|
|
---|---|---|
Owners: |
|
This resource does not have an owner who is an active HydroShare user. Contact CUAHSI (help@cuahsi.org) for information on this resource. |
Type: | Resource | |
Storage: | The size of this resource is 85.1 MB | |
Created: | Apr 16, 2020 at 3:08 p.m. (UTC) | |
Last updated: | Sep 01, 2020 at 9:47 a.m. (UTC) | |
Citation: | See how to cite this resource |
Sharing Status: | Public |
---|---|
Views: | 2122 |
Downloads: | 34 |
+1 Votes: | Be the first one to this. |
Comments: | No comments (yet) |
Abstract
This folder contains output files from Spieler et al. (2020), Automatic Model Structure Identification for Conceptual Hydrologic Models, Water Resources Research, doi: https://doi.org/10.1029/2019WR027009
Output files for a synthetic and real world experiment conducted in the paper are presented.
The data for the synthetic experiment contains:
- 100 identified AMSI models for 20 synthetic experiments for five different calibration budgets
- the objective function values (NSE) of all 100 models for all experiments & all different budgets (100*20*5)
The data for the real world experiment contains:
- 100 identified AMSI models for 12 MOPEX catchments
- the objective function values (NSE) of all 100 models for all catchments in calibration and validation
- dicharge timeseries for 100 identified AMSI models for all catchments in validation
Study abstract:
Choosing (an) adequate model structure(s) for a given purpose, catchment, and data situation is a critical task in the modelling chain. However, despite model intercomparison studies, hypothesis testing approaches with modular modelling frameworks, and continuous efforts in model development and improvement, there are still no clear guidelines for identifying a preferred model structure. By introducing a framework for Automatic Model Structure Identification (AMSI), we support the process of identifying (a) suitable model structure(s) for a given task. The proposed AMSI-framework employs a combination of the modular hydrological model RAVEN and the heuristic global optimization algorithm dynamically dimensioned search (DDS). It is the first demonstration of a mixed-integer optimization algorithm applied to simultaneously optimize model structure choices (integer decision variables) and parameter values (continuous decision variables) in hydrological modelling. The AMSI-framework is thus able to sift through a vast number of model structure and parameter choices for identifying the most adequate model structure(s) for representing the rainfall-runoff behavior of a catchment. We demonstrate the feasibility of the approach by re-identifying given model structures that produced a specific hydrograph and show the limits of the current setup via a real-world application of AMSI on twelve MOPEX catchments. Results show that the AMSI-framework is capable of inferring feasible model structures reproducing the rainfall-runoff behaviour of a given catchment. However, it is a complex optimization problem to identify model structure and parameters simultaneously. The variance in the identified structures is high due to near equivalent diagnostic measures for multiple model structures, reflecting substantial model equifinality. Future work with AMSI should consider the use of hydrologic signatures, case studies with multiple types of observation data, and the use of mixed-integer multi-objective optimization algorithms.
Subject Keywords
Coverage
Spatial
Temporal
Start Date: | |
---|---|
End Date: |
Content
Related Resources
The content of this resource references | https://linkinghub.elsevier.com/retrieve/pii/S002216940500329X |
The content of this resource is derived from | https://hydrology.nws.noaa.gov/pub/gcip/mopex/US_Data/ |
How to Cite
This resource is shared under the Creative Commons Attribution CC BY.
http://creativecommons.org/licenses/by/4.0/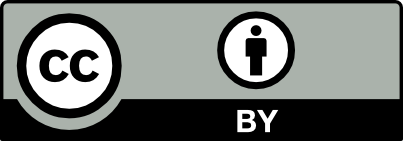
Comments
There are currently no comments
New Comment