Checking for non-preferred file/folder path names (may take a long time depending on the number of files/folders) ...
This resource contains some files/folders that have non-preferred characters in their name. Show non-conforming files/folders.
This resource contains content types with files that need to be updated to match with metadata changes. Show content type files that need updating.
Rapid inundation mapping using NWM, satellite observations, and a convolutional neural network - Demonstrated on California Atmospheric Rivers 2023
Authors: |
|
|
---|---|---|
Owners: |
|
This resource does not have an owner who is an active HydroShare user. Contact CUAHSI (help@cuahsi.org) for information on this resource. |
Type: | Resource | |
Storage: | The size of this resource is 666.3 MB | |
Created: | Aug 01, 2024 at 1:14 p.m. (UTC) | |
Last updated: | Sep 25, 2024 at 4:33 p.m. (UTC) (Metadata update) | |
Published date: | Aug 01, 2024 at 8:01 p.m. (UTC) | |
DOI: | 10.4211/hs.8b76906c4b604c458fbcb5ea7c8c0be7 | |
Citation: | See how to cite this resource |
Sharing Status: | Published |
---|---|
Views: | 1227 |
Downloads: | 45 |
+1 Votes: | Be the first one to this. |
Comments: | No comments (yet) |
Abstract
This dataset supports the analysis presented in our paper (https://agupubs.onlinelibrary.wiley.com/doi/10.1029/2024GL109424) on flood prediction using the Novel Water Model-Convolutional Neural Network (NWM-CNN). Aimed at enhancing flood forecasting and resilience, this collection encompasses a comprehensive compilation of flood events across California during the Atmospheric River events during the water year 2023. Included are NWM-CNN predictions, Sentinel-1 flood observations, and historical flood damage reports for Sacramento County, California, during the significant 2023 atmospheric river event and over the past decades.
The dataset is structured to facilitate a detailed examination of the NWM-CNN model's performance in predicting surface water area with high temporal resolution and accuracy. By integrating satellite imagery with hydrodynamic modeling, the NWM-CNN model represents a significant advancement in flood modeling, offering an effective tool for damage assessment, flood forecasting, and supporting parametric insurance solutions.
Key components of the dataset include:
* NWM-CNN Predictions: Model predictions at a 250m grid cell resolution.
* Sentinel-1 Flood Observations: Satellite-derived flood extents used for comparison against model predictions.
* Flood Damage Reports: Historical records of flood damage within Sacramento County, providing a ground-truth comparison for model efficacy.
Code to analyze this data is available here:
Jonathan Frame. (2024). jmframe/NWM_CNN_california_AR_2023: GRL Paper Proof 1 (1.0.0). Zenodo. https://zenodo.org/records/13153247. DOI: 10.5281/zenodo.13153247
By making this data publicly available, we aim to contribute to the collective efforts in reducing the impacts of climate disasters through improved access to scientific information and resources.
Subject Keywords
Coverage
Spatial
Temporal
Start Date: | |
---|---|
End Date: |
Content
Related Resources
This resource updates and replaces a previous version | Frame, J. M. (2024). NWM_CNN_California_AR_2023, HydroShare, http://www.hydroshare.org/resource/dbf8e4c2a39a4c228db867b04f9c21ed |
Credits
Funding Agencies
This resource was created using funding from the following sources:
Agency Name | Award Title | Award Number |
---|---|---|
Floodbase |
How to Cite
This resource is shared under the Creative Commons Attribution CC BY.
http://creativecommons.org/licenses/by/4.0/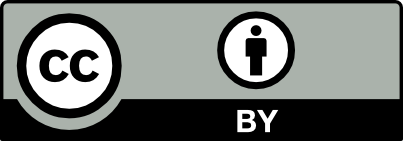
Comments
There are currently no comments
New Comment