Checking for non-preferred file/folder path names (may take a long time depending on the number of files/folders) ...
This resource contains some files/folders that have non-preferred characters in their name. Show non-conforming files/folders.
This resource contains content types with files that need to be updated to match with metadata changes. Show content type files that need updating.
Feature extraction approaches for leaf area index estimation in California vineyards via machine learning algorithms
Authors: |
|
|
---|---|---|
Owners: |
|
This resource does not have an owner who is an active HydroShare user. Contact CUAHSI (help@cuahsi.org) for information on this resource. |
Type: | Resource | |
Storage: | The size of this resource is 4.0 MB | |
Created: | Oct 25, 2021 at 4:35 p.m. | |
Last updated: | Oct 27, 2021 at 12:45 a.m. | |
DOI: | 10.4211/hs.923cf9a7a3bb49369a4e65d48237002b | |
Citation: | See how to cite this resource | |
Content types: | Geographic Feature Content Geographic Raster Content |
Sharing Status: | Published |
---|---|
Views: | 1435 |
Downloads: | 88 |
+1 Votes: | 1 other +1 this |
Comments: | No comments (yet) |
Abstract
Accurate leaf area index (LAI) estimation through machine learning (ML) algorithms is a channel for better understanding and monitoring the existing biomass and it relates to the distribution of energy fluxes and evapotranspiration partitioning. In order to support the ML algorithm for accurate LAI estimation, the supporting data (or features) gained from the sUAS platform are challenging in terms of variety, quantity, and quality. This project provides two types of feature-extraction approaches and the demo data to show how a variety of features are generated based on the sUAS platform via the python language. This project is also part of our pending paperwork. Other researchers can also use this project based on their sUAS platform to gain the features for estimation of their interested parameters, such as biomass and leaf water potential.
Subject Keywords
Coverage
Spatial
Temporal
Start Date: | |
---|---|
End Date: |
Content
Data Services
Related Resources
This resource is referenced by | https://www.ars.usda.gov/northeast-area/beltsville-md-barc/beltsville-agricultural-research-center/hydrology-and-remote-sensing-laboratory/docs/grapex/grapex-home/ |
Credits
Funding Agencies
This resource was created using funding from the following sources:
Agency Name | Award Title | Award Number |
---|---|---|
NASA | NNX17AF51G | |
Utah Water Research Laboratory | Student Fellowship |
Contributors
People or Organizations that contributed technically, materially, financially, or provided general support for the creation of the resource's content but are not considered authors.
Name | Organization | Address | Phone | Author Identifiers |
---|---|---|---|---|
Carri Richards | Utah State University | |||
Wasim Akram Khan | Utah State University |
How to Cite
This resource is shared under the Creative Commons Attribution CC BY.
http://creativecommons.org/licenses/by/4.0/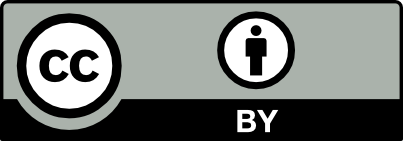
Comments
There are currently no comments
New Comment