Checking for non-preferred file/folder path names (may take a long time depending on the number of files/folders) ...
This resource contains some files/folders that have non-preferred characters in their name. Show non-conforming files/folders.
This resource contains content types with files that need to be updated to match with metadata changes. Show content type files that need updating.
An analytical solution for rapidly predicting post-fire peak streamflows for small watersheds in southern California
Authors: |
|
|
---|---|---|
Owners: |
|
This resource does not have an owner who is an active HydroShare user. Contact CUAHSI (help@cuahsi.org) for information on this resource. |
Type: | Resource | |
Storage: | The size of this resource is 26.2 KB | |
Created: | Oct 25, 2020 at 5:44 p.m. (UTC) | |
Last updated: | Dec 01, 2020 at 4:33 a.m. (UTC) (Metadata update) | |
Published date: | Dec 01, 2020 at 4:33 a.m. (UTC) | |
DOI: | 10.4211/hs.9e38375a19cf4355aac466ccd78e8282 | |
Citation: | See how to cite this resource |
Sharing Status: | Published |
---|---|
Views: | 1942 |
Downloads: | 31 |
+1 Votes: | 1 other +1 this |
Comments: | No comments (yet) |
Abstract
Following wildfires, the probability of flooding and debris flows increase, posing risks to human lives, downstream communities, infrastructure, and ecosystems. In southern California (USA), the Rowe, Countryman, and Storey (RCS) 1949 methodology is an empirical method that is used to rapidly estimate post‐fire peak streamflow. We re‐evaluated the accuracy of RCS for 33 watersheds under current conditions. Pre‐fire peak streamflow prediction performance was low, where the average R2 was 0.29 and average RMSE was 1.10 cms/km2 for the 2‐ and 10‐year recurrence interval events, respectively. Post‐fire, RCS performance was also low, with an average R2 of 0.26 and RMSE of 15.77 cms/km2 for the 2‐ and 10‐year events. We demonstrated that RCS overgeneralizes watershed processes and does not adequately represent the spatial and temporal variability in systems affected by wildfire and extreme weather events and often underpredicted peak streamflow without sediment bulking factors. A novel application of machine learning was used to identify critical watershed characteristics including local physiography, land cover, geology, slope, aspect, rainfall intensity, and soil burn severity, resulting in two random forest models with 45 and five parameters (RF‐45 and RF‐5, respectively) to predict post‐fire peak streamflow. RF‐45 and RF‐5 performed better than the RCS method; however, they demonstrated the importance and reliance on data availability. The important parameters identified by the machine learning techniques were used to create a three‐dimensional polynomial function to calculate post‐fire peak streamflow in small catchments in southern California during the first year after fire (R2 = 0.82; RMSE = 6.59 cms/km2) which can be used as an interim tool by post‐fire risk assessment teams. We conclude that a significant increase in data collection of high temporal and spatial resolution rainfall intensity, streamflow, and sediment loading in channels will help to guide future model development to quantify post‐fire flood risk.
Subject Keywords
Coverage
Spatial
Temporal
Start Date: | |
---|---|
End Date: |
Content
Additional Metadata
Name | Value |
---|---|
Supplementary Information | Additional details on each of the features of this dataset can be found in the "Supplementary Information " section of the published paper (https://onlinelibrary.wiley.com/doi/abs/10.1002/hyp.13976) |
Credits
Funding Agencies
This resource was created using funding from the following sources:
Agency Name | Award Title | Award Number |
---|---|---|
Joint Fire Science Program | Graduate Research Innoation Award | 19-1-01-55 |
San Diego State University | Master's Research Scholarship | |
Department of Conservation - California Geological Survey | Graduate Assistant - Geology, Hydrology, Geomorphology (San Diego) |
How to Cite
This resource is shared under the Creative Commons Attribution CC BY.
http://creativecommons.org/licenses/by/4.0/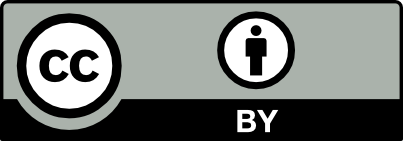
Comments
There are currently no comments
New Comment