Checking for non-preferred file/folder path names (may take a long time depending on the number of files/folders) ...
This resource contains some files/folders that have non-preferred characters in their name. Show non-conforming files/folders.
This resource contains content types with files that need to be updated to match with metadata changes. Show content type files that need updating.
Authors: |
|
|
---|---|---|
Owners: |
|
This resource does not have an owner who is an active HydroShare user. Contact CUAHSI (help@cuahsi.org) for information on this resource. |
Type: | Resource | |
Storage: | The size of this resource is 432.0 MB | |
Created: | May 06, 2020 at 1:22 p.m. (UTC) | |
Last updated: | Feb 06, 2024 at 2:50 p.m. (UTC) (Metadata update) | |
Published date: | May 06, 2020 at 2:10 p.m. (UTC) | |
DOI: | 10.4211/hs.9f981ae4e68b4f529cdd7a5c9013e27e | |
Citation: | See how to cite this resource | |
Content types: | File Set Content Single File Content |
Sharing Status: | Published |
---|---|
Views: | 4264 |
Downloads: | 727 |
+1 Votes: | Be the first one to this. |
Comments: | No comments (yet) |
Abstract
Soil moisture is key for quantifying soil-atmosphere interactions and the ESA-CCI (European Space Agency-Climate Change Initiative) provides historical (>30 years) satellite soil moisture global grids with spatial resolution of ~27km. This dataset is incomplete (contains gaps) due to conditions such as dense vegetation or extremely dry surfaces. Here we provide a framework to increase the spatial resolution and fill gaps (reporting associated uncertainty) of the ESA-CCI (v4.5) soil moisture dataset. The outcome is a new dataset of gap-free global mean annual soil moisture and uncertainty
for 28 years (1991-2018) across 15km grids. We compare the performance of machine learning odels using only terrain parameters (e.g., slope, wetness index) against predictions using terrain parameters, bioclimatic information, and soil type classes. We use independent field information from the International Soil Moisture Network (ISMN, n=13376) and in-situ precipitation records (n=171) only for model evaluation purposes. Using only terrain parameters to predict soil moisture results in a parsimonious approach comparable with a more complex model that includes additional bioclimatic and soil information. The correlation between observed and predicted soil moisture values varies from r=0.69 to r=0.87 with root mean squared errors (RMSE) around 0.03 and 0.04 m3/m3. Our soil moisture predictions improve: (a) the correlation with the ISMN (when compared with the original ESA-CCI product) from r=0.30 (RMSE=0.09 m3/m3 ) to r=0.66 (RMSE=0.05 m3/m3 ); and (b) the
correlation with local precipitation records across boreal (from r=<0.3 up r=0.49) or tropical areas (from r=<0.3 to r=0.46) which are currently poorly represented in the ISMN. Temporal trends show a decline of global annual soil moisture using: a) data from the ISMN (-1.5 [-1.8, -1.24]%, b) associated locations from the original ESA-CCI dataset (- 0.87[-1.54, -0.17]%), c) associated locations from predictions based on terrain parameters (-0.85[-1.01, -0.49]%), and d)associated locations from predictions including bioclimatic and soil type classes (-0.68[-0.91, -0.45]%). Our parsimonious downscaled soil moisture predictions are independent of climate variables and vegetation indexes, to avoid potential spurious correlations in future research, and they complement information about soil moisture dynamics worldwide.
Subject Keywords
Coverage
Spatial
Temporal
Start Date: | |
---|---|
End Date: |
Content
readme.txt
This folder contains the information required to reproduce the soil moisture predictions and the cross validation results presented in Guevara, Taufer and Vargas 2019, Gap-Free Global Annual Soil Moisture: 15km Grids for 1991-2016 (in review).The training soil moisture dataset used in this study is available (here: https://www.esa-soilmoisture-cci.org/) thanks to the ESA-CCI soil moisture initiative. We provide two soil moisture annual predictions (1991-2018). The fist model predictions are based on terrain parameters (sm_kknn_terrain.tar.xz) (calculated on SAGA GIS using elevation data from https://www2.jpl.nasa.gov/srtm/) and the second model predictions include bioclimatic data (from http://www.fao.org/nr/gaez/en/) and soil type classes (from https://daac.ornl.gov/SOILS/guides/HWSD.html) as prediction factors (sm_kknn_eco_swc_terrain_15km..tar.xz). Each set of soil moisture predictions are delivered in independent folders. A model was generated for each year and on each folder there are accuracy reports for each model/year. Accuracy was calculated using 10-fold cross-validation. We also provide soil moisture field data (from https://ismn.geo.tuwien.ac.at/en/)organized in an annual basis. This dataset is provided in a native R format *.rds. In this same format *.rds we also provide the organized set of prediction factors and a illustrative figure file *.pdf showing the data and results. All the code to reproduce these soil moisture predictions is also available here: https://github.com/vargaslab/Global_Soil_Moisture.
Related Resources
The content of this resource is derived from | For soil type classes: https://daac.ornl.gov/SOILS/guides/HWSD.html |
The content of this resource is derived from | For soil moisture: https://www.esa-soilmoisture-cci.org/node/137 |
The content of this resource is derived from | For terrain parameters: http://www.saga-gis.org/ |
The content of this resource is derived from | The source DEM: https://topex.ucsd.edu/sandwell/publications/124_MG_Becker.pdf |
The content of this resource is derived from | For statistical computing: https://www.r-project.org/ |
The content of this resource is derived from | Example with higher resolution (e.g., CONUS 1km) https://journals.plos.org/plosone/article?id=10.1371/journal.pone.0219639 |
The content of this resource is derived from | For Agro-ecological data http://www.fao.org/nr/gaez/en/ |
This resource updates and replaces a previous version | Guevara, M., R. Vargas, M. Taufer (2022). Gap-Free Global Annual Soil Moisture: 15km Grids for 1991-2016, HydroShare, http://www.hydroshare.org/resource/7ae32f5d66e54c6a890bb4b14c643d15 |
Credits
Funding Agencies
This resource was created using funding from the following sources:
Agency Name | Award Title | Award Number |
---|---|---|
National Science Foundation | CIF21 DIBBs: PD: Cyberinfrastructure Tools for Precision Agriculture in the 21st Century | 1724847 |
Mexican National Council for Science and Technology (CONACyT) | PhD Fellowship | 382790 |
How to Cite
This resource is shared under the Creative Commons Attribution CC BY.
http://creativecommons.org/licenses/by/4.0/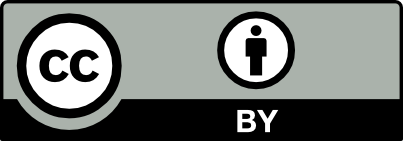
Comments
There are currently no comments
New Comment