Checking for non-preferred file/folder path names (may take a long time depending on the number of files/folders) ...
This resource contains some files/folders that have non-preferred characters in their name. Show non-conforming files/folders.
This resource contains content types with files that need to be updated to match with metadata changes. Show content type files that need updating.
Annual soil moisture predictions across conterminous United States using remote sensing and terrain analysis across 1 km grids (1991-2016)
Authors: |
|
|
---|---|---|
Owners: |
|
This resource does not have an owner who is an active HydroShare user. Contact CUAHSI (help@cuahsi.org) for information on this resource. |
Type: | Resource | |
Storage: | The size of this resource is 2.7 GB | |
Created: | Jun 20, 2019 at 4:01 p.m. | |
Last updated: | Mar 23, 2020 at 11:16 p.m. | |
DOI: | 10.4211/hs.b8f6eae9d89241cf8b5904033460af61 | |
Citation: | See how to cite this resource | |
Content types: | File Set Content Single File Content Geographic Raster Content |
Sharing Status: | Published |
---|---|
Views: | 4505 |
Downloads: | 4370 |
+1 Votes: | 1 other +1 this |
Comments: | 1 comment |
Abstract
We provide 26 annual soil moisture predictions across conterminous United States for the years 1991-2016. These predictions are provided in raster files with a geographical (lat, long) projection system and a spatial resolution of 1 x 1 km grids (folder: soil_moisture_annual_grids_1991_2016). These raster files were populated with soil moisture data based on multiple kernel based machine learning models for coupling hydrologically meaningful terrain parameters (the explanatory variables) with soil moisture microwave records (the response variable) from the European Space Agency Climate Change Initiative. We provide a raster stack with the annual training data from satellite soil moisture estimates (file: annual_means_of _ESA_CCI_soil_moiture_1991_2016.tif) and the explanatory variables (terrain) calculated on SAGA GIS (System of Automated Geoscientific Analysis) using digital terrain analysis (folder: explanatory_variables_dem). The explained variance for all models-years was >70% (10-fold cross-validation). The 1 km soil moisture grids (compared to the original satellite soil moisture estimates) had higher correlations with field soil moisture observations from the North American Soil Moisture Database (n=668 locations with available data between 1991-2013; 0-5 cm depth) than soil moisture microwave records. For further information refer to our preprint in bioRxiv: https://www.biorxiv.org/content/biorxiv/early/2019/07/01/688846.full.pdf
Subject Keywords
Coverage
Spatial
Temporal
Start Date: | |
---|---|
End Date: |
Content
README.txt
This folder contains the information required to reproduce the soil moisture predictions and the cross valdiation results presented in Guevara and Vargas 2019, Downscaling satellite soil moisture using geomorphometry and machine learning (in review Plos one). The folder soil_moisture_annual_grids_1991_2016 contains the annual predictions of soil moisture across conterminous United States in 1x1 km grids. These files are provided in generic *.tif raster files. The folder explanatory_variables_dem contains the explanatory variables (predictors) used for generating the soil moisture 1 km grids. These files represent multiple terrain features and they were calculated in SAGA GIS. These files are provided in a raster native format of SAGA GIS (*.sgrd). These files are described in a document table with the file in the same folder (explanatory_variables_description_SAGA_GIS_1km.docx). The values of soil moisture estimates from the European Space Agency Climate Change Initiative were averaged in an annual basis. This information was used as training data for the machine learning model using the aforementioned terrain parameters as prediction factors. This file has a spatial resolution of >25 km grids. We provide a raster stack containing the annual soil moisture estimates used for trainig the models in a generic raster stack *.tif file (annual_means_of _ESA_CCI_soil_moiture_1991_2016.tif). All analysis were performed in R. We also provide the R code for generating and validating the soil moisture predictions across CONUS (guevara_vargas_soil_moisture_geomorphometry_kknn.R).
Data Services
Related Resources
The content of this resource is derived from | For soil moisture: https://www.esa-soilmoisture-cci.org/node/137 |
The content of this resource is derived from | For terrain parameters: http://www.saga-gis.org/ |
The content of this resource is derived from | The source DEM: https://topex.ucsd.edu/sandwell/publications/124_MG_Becker.pdf |
The content of this resource is derived from | For statistical computing: https://www.r-project.org/ |
The content of this resource is derived from | Plos ONE paper: https://journals.plos.org/plosone/article?id=10.1371/journal.pone.0219639 |
This resource has been replaced by a newer version | Guevara, M., R. Vargas (2020). Annual soil moisture predictions across conterminous United States using remote sensing and terrain analysis across 1 km grids (1991-2016), HydroShare, http://www.hydroshare.org/resource/fb83dc83bdd0452782d622dfb38e67f2 |
Credits
Funding Agencies
This resource was created using funding from the following sources:
Agency Name | Award Title | Award Number |
---|---|---|
National Science Foundation | CIF21 DIBBs: PD: Cyberinfrastructure Tools for Precision Agriculture in the 21st Century | 1724847 |
Mexican National Council for Science and Technology (CONACyT) | PhD Fellowship | 382790 |
Contributors
People or Organizations that contributed technically, materially, financially, or provided general support for the creation of the resource's content but are not considered authors.
Name | Organization | Address | Phone | Author Identifiers |
---|---|---|---|---|
Michela Taufer | University of Tennessee | Min H. Kao Building, Room 620 1520 Middle Drive Knoxville, TN 37996-2250 | 865-974-9952 | GoogleScholarID |
How to Cite
This resource is shared under the Creative Commons Attribution CC BY.
http://creativecommons.org/licenses/by/4.0/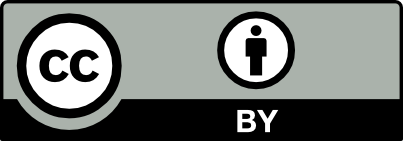
Comments
Mario Guevara 4 years, 5 months ago
PEER REVIEWED PUBLICATION OF THIS RESOURCE AVAILABLE AT PLOS ONE:
Replyhttps://journals.plos.org/plosone/article/metrics?id=10.1371/journal.pone.0219639
New Comment