Checking for non-preferred file/folder path names (may take a long time depending on the number of files/folders) ...
This resource contains some files/folders that have non-preferred characters in their name. Show non-conforming files/folders.
This resource contains content types with files that need to be updated to match with metadata changes. Show content type files that need updating.
Authors: |
|
|
---|---|---|
Owners: |
|
This resource does not have an owner who is an active HydroShare user. Contact CUAHSI (help@cuahsi.org) for information on this resource. |
Type: | Resource | |
Storage: | The size of this resource is 2.5 GB | |
Created: | Jul 12, 2023 at 8:03 p.m. (UTC) | |
Last updated: | Jul 12, 2023 at 11:05 p.m. (UTC) | |
Citation: | See how to cite this resource | |
Content types: | Multidimensional Content |
Sharing Status: | Public |
---|---|
Views: | 1598 |
Downloads: | 4 |
+1 Votes: | Be the first one to this. |
Comments: | No comments (yet) |
Abstract
Snow is a vital component of the global land surface energy and water budget. In this study, we investigate the how synthetic observations of snow water equivalent (SWE) representative of a synthetic aperture radar remote sensing platform could improve spatiotemporal estimates of snowpack. We use an Observation System Simulation Experiment, specifically investigating how much snow simulated using popular models and forcing data could be improved by assimilating synthetic observations of SWE. We focus this study across a 24°-by-37° domain in the Western United States and Canada, simulating snow at approximately 250 m resolution and hourly timesteps in water-year 2019. We perform two data assimilation experiments, including: 1) a simulation excluding synthetic observations in forests where canopies obstruct remote sensing retrievals, and 2) a simulation inferring snow distribution in forested grid cells using synthetic observations from nearby canopy-free grid cells. Results found that assimilating synthetic SWE observations improved average SWE biases at peak snowpack timing in shrub, grass, crop, bare-ground, and wetland land cover types from 14%, to within 1%. However, forested grid cells contained a disproportionate amount of SWE volume. In forests, SWE mean absolute errors at peak snowpack were 111 mm, and average SWE biases were on the order of 150%. Here, the data assimilation approach that estimated forest SWE using observations from the nearest canopy-free grid cells substantially improved these SWE biases (18%) and the SWE mean absolute error (27 mm). Data assimilation also improved estimates of the temporal evolution of both SWE, even in spring snowmelt periods when melting snow and high snow liquid water content block the synthetic SWE retrievals. In fact, in the Upper Colorado River basin, melt-season SWE biases were improved from 63% to within 1%, and the Nash Sutcliffe Efficiency of runoff improved from –2.59 to 0.22. These results demonstrate the value of a snow-focused globally relevant remote sensing platform, and data assimilation for improving the characterization of SWE and associated water availability.
Subject Keywords
Coverage
Spatial
Temporal
Start Date: | |
---|---|
End Date: |
Content
README.txt
README file containing descriptions for data corresponding with Pflug et al. (2023) "Extending the utility of space-borne snow water equivalent observations over vegetated areas with data assimilation" Data last updated: J. Pflug (justin.pflug@nasa.gov) -- 12 Jul 2023 -domain_params.nc [Land Data Toolkit file]: - Static data for the modeling domain investigated in this study. Variables include: - lat: Latitude (degrees North, WGS84) for each model grid cell - lon: Longitude (degrees East, WGS84) for each model grid cell - ELEVATION: Domain elevation (m) based on the National Elevation Dataset (NED; https://gdg.sc.egov.usda.gov/Catalog/ProductDescription/NED.html) - LANDCOVER: Domain integer landcover map, based on the landcover map from IGBP/NCEP (e.g., http://www.eomf.ou.edu/static/IGBP.pdf) The following files are spatial outputs on the grid specified by the lat/lon grid from "domain_params.nc" above. Due to the large number of grid cells, files are saved in ascii format. - huc_basins.asc: Map of integers representing the grid cells that fell within each of the subbasins analyzed in this study. Basins are labeled as the following: - 1: Upper Colorado - 2: Great Basin - 3: Pacific Northwest - 4: California - *_DJF_SWE.asc: Maps of average winter (December, January, February) SWE for each simulation, rounded to the nearest mm. Filename prefixes represent the simulation. They are as follows: - NR: Nature run - OL: Open loop - DA1: Simulation with data assimilation, without the forest strategy - DA2: Simulation with data assimilation, with the forest strategy - *_20190313_SWE.asc: Maps of SWE (rounded to the nearest mm) on Mar 13 2019. The file prefixes reference the simulation and are identical to the previous dataset. The remainder of the files are temporal outputs aggregated across the grid cells that fall within each of the huc basins (e.g., from "huc_basins.asc"). Each entry represents variables at daily timesteps between 1 October 2018 and 15 Aug 2019. - *A*_temporal_*B*_HUC*C*.asc --> wildcards represent the following: - *A*: The simulation (NR, OL, DA1, or DA2) - *B*: The output variable: - SWE_tavg: SWE averaged at daily timesteps across all grid cells within a given basin. Outputs are rounded to the nearest mm - Qtot_tavg: Total runoff summed at daily timesteps across all grid cells within a given basin. Outputs are rounded to the nearest m - *C*: The subbasin, identical to those listed above (e.g., Upper Colorado, California, etc.)
Data Services
How to Cite
This resource is shared under the Creative Commons Attribution CC BY.
http://creativecommons.org/licenses/by/4.0/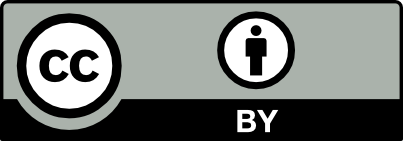
Comments
There are currently no comments
New Comment